On mathematical publishers and AI partnerships
A text prepared for the 2025 Joint Mathematics Meetings in Seattle
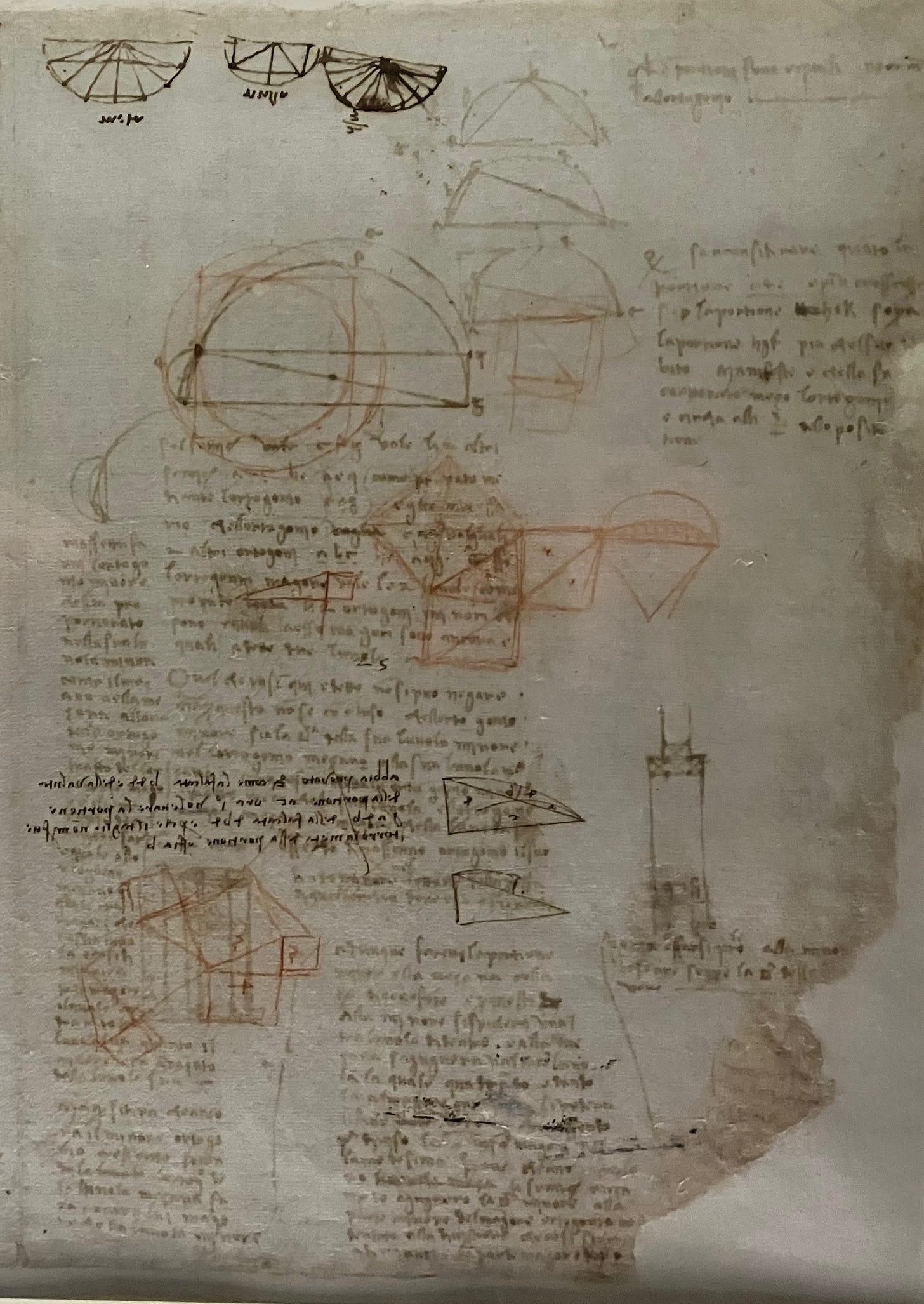
None of the four factors [in fair use] seem to weigh in favor of ChatGPT being a fair use of its training data. That being said, none of the arguments here are fundamentally specific to ChatGPT either, and similar arguments could be made for many generative AI products in a wide variety of domains.
(Suchir Balaji, 10/23/24, less than two months before his suicide.)
This post continues the discussion begun when I learned about Google’s possible infringement of mathematicians’ copyrights and continued when the news came out that scientific publishers had begun signing deals to license their data for training. A few months ago I was invited to participate in an
AMS Committee on Publications Panel Discussion: Artificial Intelligence and Publishing
at the 2025 Joint Mathematics Meeting in Seattle, with the following description:
This panel discussion will survey the effects of artificial intelligence on mathematical publishing, with an eye towards issues directly affecting AMS and its members.
I prepared a presentation of my opinions on such licensing deals, only to learn that the speakers
Robert M Harington, American Mathematical Society
Michael Harris, Columbia University
Emily Riehl, Johns Hopkins University
Marc Strauss, Springer Nature
Ramin Zabih, Cornell
would each be given 5 minutes at the outset to make our points, before the session would be thrown open to public questions and comments.
So I am making the full presentation available here ahead of the meeting. I begin with a series of brief quotes from articles published last summer in The Bookseller, after the first “AI partnerships” between publishers and the tech industry were announced.
A July 19 article opened with the following paragraph:
Authors have expressed their shock after the news that academic publisher Taylor & Francis, which owns Routledge, had sold access to its authors’ research as part of an Artificial Intelligence (AI) partnership with Microsoft—a deal worth almost £8m ($10m) in its first year.
The following week a second article reported that T&F was “set to make £58m” in 2024 from Microsoft and a second partnership, which it “declined to name”:
These partnerships include data access agreements Taylor & Francis archive content "to help train and improve the relevance of outputs from Large Language Models (LLMs)".
It described these as "a source of significant new value for Taylor & Francis and additional royalties for authors, with total AI partnership revenues expected to be over $75m in 2024".
At the time of publication, authors had heard nothing about these “additional royalties.”
The Bookseller followed up on August 1, asking “a range of publishers across both academic and corporate, whether their authors’ work was being used for AI research.” The article quotes Sara Lloyd, group communications director and global AI lead for Pan Macmillan: “We have not sold access to copyright works for AI purposes… we understand from the round-table events we’ve hosted with authors, illustrators, agents and industry bodies that using copyright works to train underlying large language models (LLMs) is a particular concern.” The article continues:
Hachette and HarperCollins also confirmed they had not sold any material to AI for research. “We have not sold any access for AI research," a HarperCollins spokesperson told The Bookseller. "If we were to reach an agreement to do so, we would provide authors the option of whether or not to participate.”
Oxford University Press, Cambridge University Press, and Wiley, on the other hand, all reported on deals with unidentified tech corporations, while Pearson “declined to comment.” Cambridge was the only AI partner to describe protections for authors:
“We are giving our authors and partners the choice to opt in to future licensing agreements with generative AI providers,” Mandy Hill, managing director of academic publishing at CUP said.
“We will put authors’ interests and desires first, before allowing their work to be licensed for GenAI. We believe that AI technologies have opportunities and risks for scholarly content. Where Cambridge-published content is used, it must be properly attributed, licensed, founded on permissions and with fair renumeration for both authors and publishers.”
Wiley had already announced a “revolutionary evolution” in 2021 in a pamphlet, which you can download on this page, entitled
What does the future hold, and how will we get there?
Writing and synthesizing ideas is an important part of research, but as long as we make researchers send us word documents and static images as opposed to data, code and detailed methods, we are “dumbing down” the essential artifacts that support the conclusions.
Wiley announced its AI partnerships, and specificially its partnership with an “AI research assistant” called Potato, in an October 17 press release, entitled
Wiley Launches New Partnership Innovation Program to Deliver AI Advantages for Researchers and Practitioners
The leader of Wiley’s AI Growth team equivocated with regard to the “advantages” when a reporter for The Scholarly Kitchen asked
There is discussion about if and how authors and copyright holders should opt their works in or out of deals with AI model developers. What is the role of authors’ interests/ expectations in this process?
Readers can judge whether or not authors should feel reassured by Wiley’s response:
The commitment we make when we sign contracts with our authors and other copyright holders, such as our society publishing partners, is to ensure that their interests are safeguarded in a rapidly evolving digital landscape. AI and LLMs are just two of the most recent developments in what has been a hugely dynamic period in publishing. Most agreements with authors and copyright holders include broad dissemination rights across formats consistent with this shared mission.
We’ve seen claims that AI model developers can rely on fair use to use content without needing to license it. Among other flaws with these claims, when there is a robust marketplace for licensing, the argument for fair use fails. By establishing a clear and structured marketplace for licensing, we’re not only protecting the interests of authors and copyright holders, but we’re also safeguarding the very concept of copyright itself.
Readers who are concerned by the prospect of similar licensing agreements by mathematical publishers are encouraged to look up the articles linked above, either before or after reading the text I prepared for the JMM panel:
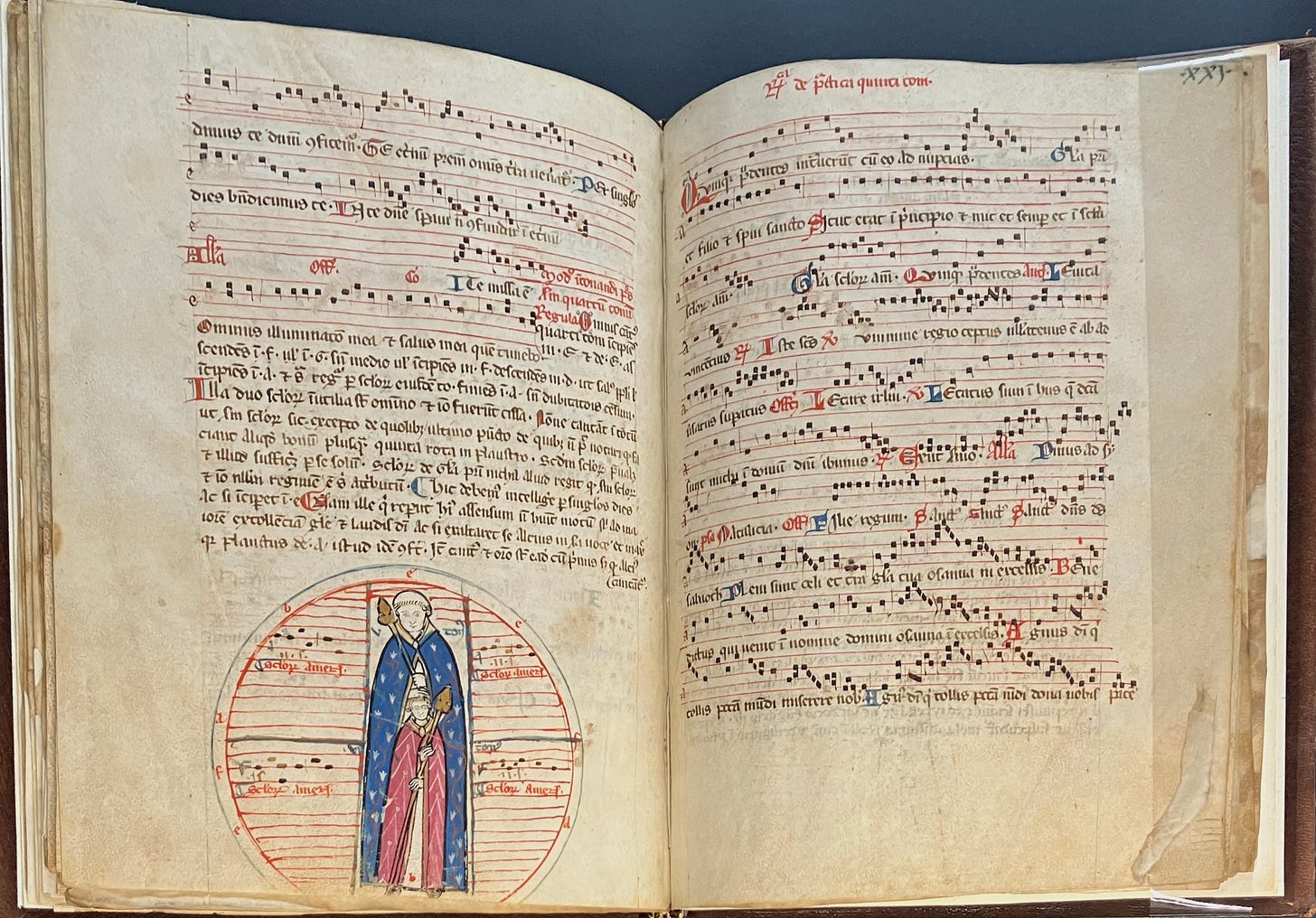
In what follows I identify three possible attitudes to the proposal to license the mathematical corpus as training data: enthusiasm, acquiescence, and hostility. None of the three is an accurate characterization of my own perspective, which is expressed in all its ambiguity and ambivalence in my texts on this site. What I find most perplexing is that, in this debate as in all the others concerning mechanization of mathematics, the fact that different attitudes are possible is not acknowledged, except in the form of caricature: for or against progress. My main objective in spending so much time talking and writing about these issues is to change this situation, to convince colleagues that what is at stake has to do with democracy and power and is not determined impersonally by the evolution of technology.
I take heart from the knowledge that in every other comparable domain, whether in the arts or academia, there is a very lively debate and a deep distrust of the industry. Thus, when I mentioned this panel to Kate Crawford, co-founder of AI Now, after an event in New York on AI and intellectual property in the arts, her immediate reaction was that handing over the mathematical corpus to the industry was a terrible idea. Why should mathematicians think differently?
Enthusiasm. This is easy to understand. Most mathematicians are eager to expand the field, to know the answers to unsolved problems, to find new ways of thinking. The technology promises a dramatic acceleration in all these areas. Combined with the belief that only the industry has the resources to pilot this acceleration, many of the colleagues who think about this at all believe that mathematics will gain enormously by piggybacking on the industry's business plan. So by making the mathematical corpus freely available to the industry, in exchange for a modest one-time cash payment, such colleagues may expect that the industry will provide mathematics with the technical means, in the form of trained generative AI, to realize much more of its potential.
Some colleagues have also heard rumors that the industry controls fantastic wealth on a scale never before seen in history, and may believe that by rubbing shoulders with the tech titans some of this wealth will rub off on them. However, before proceeding, I should confess that I have yet to meet a single mathematician who is genuinely enthusiastic about the prospect of licensing published mathematics as training data.
The beliefs of this first — hypothetical — group of colleagues are only plausible if one assumes that the industry will preserve the values of the mathematical community. The history of mathematics should remind us that these values are more flexible and variable than we tend to believe, and could under evolve to be aligned with those of the industry, rather than vice versa. Is this desirable? The real decision-makers, the people like Sam Altman, not to mention Elon Musk, may have emphatically spoken in favor of beneficial AI, but their recent actions have just as emphatically left the impression that the industry is not to be trusted. This is simply because, like any industry, Silicon Valley is primarily a source of profits for investors. Whenever you read a Nature article about the latest supposed milestone reached by a team of mathematicians working with the industry, please remember that what you are reading is a public relations operation, primarily intended as an entry in the scramble for investment, and that the actual mathematical content is irrelevant to the industry except when it specifically applies to the technology of AI itself.
Here are a few thoughts to temper this (hypothetical) enthusiasm:
The argument is repeatedly made, on purely technical grounds, that in order for mathematics to realize the benefit of generative AI, the mathematical corpus has to be expanded by a factor of roughly 2^16, and only the industry has the capacity to realize such an expansion.
My naive thoughts about the dynamics of statistical recombination lead me to wonder whether mathematics inbred over 16 generations would really represent an improvement; the paper "Hallucinating Law" published by Stanford a year ago suggests that the result would more likely be to dilute and degrade the corpus. But my mental model is obviously simplistic and it's a really interesting philosophical as well as sociological problem to try to understand the mathematical corpus as a whole.
It's also potentially an interesting research question: is there a better model than generative AI? This is of immediate interest for at least two reasons:
1. The cost of training LLMs is prohibitive; only 4 corporations in US have enough GPUs. Those who sincerely believe that AI trained on existing mathematics will be beneficial to the discipline should be taking the time to think about whether this isn't more likely on the basis of smaller models designed and controlled by the "we" who "decide."
2. There is also a strong ethical argument against generative AI that has been completely absent from the discussions I've seen. This includes, for example, those organized by NASEM, or at IPAM in 2023, and sponsored by NSF. Why are mathematicians not talking about the environmental impact of training these systems? I'm thinking of the CO2 emissions, of course, as well as the consumption of water resources, and I assume everyone has read about Microsoft's plans to restart the Three Mile Island nuclear plant. AI is the "official theme" of this year's meetings and is the explicit theme of at least 64 panels. Mathematicians are competent to analyze the environmental implications of the technology; why is this not a theme of any of the panels?1
Acquiescence. I've encountered several versions of this attitude, but it comes down to a very simple calculation. In an ideal world it would indeed be true that "we decide our future"; but in this world "they" are much bigger and more powerful than "we" are, and it would be wise to accept their offer, and perhaps to try to negotiate a better offer, since soon enough they are likely to take our data for free and there's nothing we can do about it.
That's actually not literally true. Publishers are taking the industry to court and asking for damages. European countries and institutions are imposing strict regulations. You may wonder: how can mathematics compete in this arena? Suppose we offered to join some of these lawsuits and regulatory processes; why would anyone care? My answer is that mathematics may be lacking the material resources but continues to enjoy tremendous prestige, much more than we tend to realize. By devoting sufficient attention to the question acting in concert with other professional associations, such as the EMS, the AMS can actually transform the slogan "We decide the future" into something more than wishful thinking.
Hostility. Please don't confuse hostility to a tech industry takeover of mathematics with hostility to technology as such. If it is widely believed that the future of mathematics is bound up with AI to a degree difficult to predict — and current trends seem to suggest that this belief is widely held, though it's also difficult to determine how many mathematicians care about this at all — nothing forces us to accept that this evolution must inevitably be realized on the industry's terms. None of the speakers I've heard on this topic over the past few years has acknowledged the divergence between the interests of the industry and of mathematicians. Or rather, none of the mathematicians has acknowledged this; the computer scientists I've heard tend to take this for granted, and it's impossible to keep up with all the essays and articles and books and blogs that warn, often in very powerful language, against accepting the industry's language, priorities, and domination. (See the July 19 article in The Bookseller for examples.)
Recommendations for action
Mathematics has not evolved according to a predetermined plan. Its history has been deeply contingent and serendipity deserves to be recognized as its guiding principle. Is this a good thing? A moment's reflection will convince us that serendipity does not make for a good business plan. But mathematics is not a business, nor is it a branch of a business. For me the question of AI and mathematics publishing is just one aspect of the overriding question of whether we want this to remain true.
However, reliance on serendipity is not a substitute for effective political action. Therefore, I propose the following Recommendations. (formulated in consultation with Kate Crawford, and upon reading Sue Halpern's article The Coming Tech Autocracy in a recent issue of NYRB).2
1. Two years ago, at a meeting at IPAM, Tony Wu, now with xAI but then with Google, gave the following rationale for scraping the entirety of online mathematics in order to train a model called Minerva:
If you have the money to train the model, then go ahead
Defenders of the discipline should not allow such attitudes to go unchallenged! If an article has been posted under a no-commercial-use license, then it should be strictly off limits to the industry. If mathematical publishers do succumb to the temptation to sell out to the industry, it should only be on the basis of an opt-in model, as in the contract Cambridge University Press signed for its books.
2. Funding. Assuming an AI revolution is desirable, can mathematics carry it out on its own, and on its own terms? I'm aware of no discussion of the resources that would be necessary for such a process, but the institutional structures do exist. If the profession decides — in keeping with the banner heading for the JMM meeting — that training machines on the mathematical corpus is desirable, it should find ways to do it on its own, rather than relinquishing control to the tech industry. With a small amount of philanthropic and public funding — say $10-20 million — would it be possible to design models that could benefit mathematics? There are some recent precedents. Last month Danqi Chen gave a lecture at the Columbia Engineering School — I only learned about this weeks later — with the title
Training Language Models in Academia: Research Questions and Opportunities
Chen observes in her abstract that the development of LLMs
has been predominantly concentrated within large technology companies due to substantial computational and proprietary data requirements.
and presents an alternative
vision for how academic research can play a critical role in advancing the open language model ecosystem, particularly by developing smaller yet highly capable models and advancing our fundamental understanding of training practices.
Experts will be able to judge whether these examples Chen presents — she claims the examples she has developed with colleagues “illustrate how academic research can push the boundaries of model efficiency, capability, and scalability” — are at all relevant to mathematics.
(Meanwhile, the site Cairn.info, which provides access to a library of 1.5 million academic articles in French, is independently developing three AI-based projects at low cost, using open source software and no more than three GPUs. One project appears to be for an interactive semantic search3 of their library, of the kind that a number of mathematicians have mentioned as motivation for integrating AI. Interestingly, they place an emphasis on the role of the specific author rather than the abstract content. Large language models notoriously provide a statistical synthesis of their training data and do not systematically record the authors of the material in their training set. Is the author’s identity less important in mathematics than in the humanities and social sciences? I expect to return to this issue in a future more extensive report on Cairn’s AI projects.4)
3. Halpern's article reviews (among other books) the recent Taming Silicon Valley by Gary Marcus. In Halpern’s words,
Marcus details the demands that citizens should make of their governments and the tech companies.
It would be appropriate for mathematicians to make the same demands:
They include transparency on how AI systems work; compensation for individuals if their data is used to train LLMs and the right to consent to this use; and the ability to hold tech companies liable for the harms they cause by eliminating Section 230, imposing cash penalties, and passing stricter product liability laws, among other things. Marcus also suggests—as does Rus—that a new, AI-specific federal agency, akin to the FDA, the FCC, or the FTC, might provide the most robust oversight. As he told the Senate when he testified in May 2023:
The number of risks is large. The amount of information to keep up on is so much…. AI is going to be such a large part of our future and is so complicated and moving so fast…[we should consider having] an agency whose full-time job is to do this.
To conclude, a quotation from Kate Crawford:
The practices of data extraction and training dataset construction are premised on a commercialized capture of what was previously part of the commons. This particular form of erosion is a privatization by stealth, an extraction of knowledge value from public goods. … The new AI gold rush consists of enclosing different fields of human knowing, feeling, and action—every type of available data—all caught in an expansionist logic of never-ending collection. It has become a pillaging of public space.5
From NPR, July 12, 2024: The data centers in Northern Virginia will need the equivalent of enough energy to power 6 million homes by 2030, according to the Washington Post.
Sue Halpern, “The Coming Tech Autocracy,” New York Review of Books, November 7, 2024
For example, you can state a lemma you might need, in natural language, and ask: has anyone already proved this? You might think this is more difficult in mathematics than in the social sciences and humanities, the subjects in cairn.info’s database; but perhaps not.
I expect to be well informed on the advancement of these projects because Jean-Baptiste de Vathaire, Cairn’s Directeur général, is my brother-in-law.
Kate Crawford, Atlas of AI, p. 120.
"It has become a pillaging of public space." The pillaging of a resource is supposed to harm it, make it scarce, and negatively affect its quality and/or abundance. Where is the harm, in this case? People have been paying for the use of original material for centuries; how is the situation of tech companies paying publishers different? It's just a matter of negotiating the conditions. Where's the ethical side of it? Individual authors should be given a right to opt out but, if they are sufficiently compensated, I can't see why they would, other than for purely ideological irrational reasons (which are obvious in Harris's references to a minuscule marginal extra energy consumption of training an LLM that also includes math data or to the restarting a nuclear power plant, as if it were a bad thing no matter what. Meanwhile, we are looking at a renaissance of this clean industry that's been tragically neglected over the last decades). It's "big tech bad, rich bad, Musk bad, profit bad" ethos; historically, it hasn't served humanity well.
I am a mathematician who worked in publishing starting in 1974. I worked with Martin Gardner from 1974 until his death in 2010. While he and I were working on second editions of his Mathematical Games books Macmillan and Scientific American asserted claims to control this material, contrary to the arrangements set up by Gerard Piel and Dennis Flanagan when Martin wrote his columns and books. Despite substantial evidence from many directly involved, Doug Hofstadter, Ian Stewart, Jonathan Piel and others Macmillan and Holtzbrinck refused to acknowledge Martin’s rights and to pass on to him his share of the money they collected from sales they made of those rights without his knowledge. You may be sure this pattern will be followed by those selling rights to materials for training generative AI — only the damage will be different and far greater. I have lived it. Peter Renz