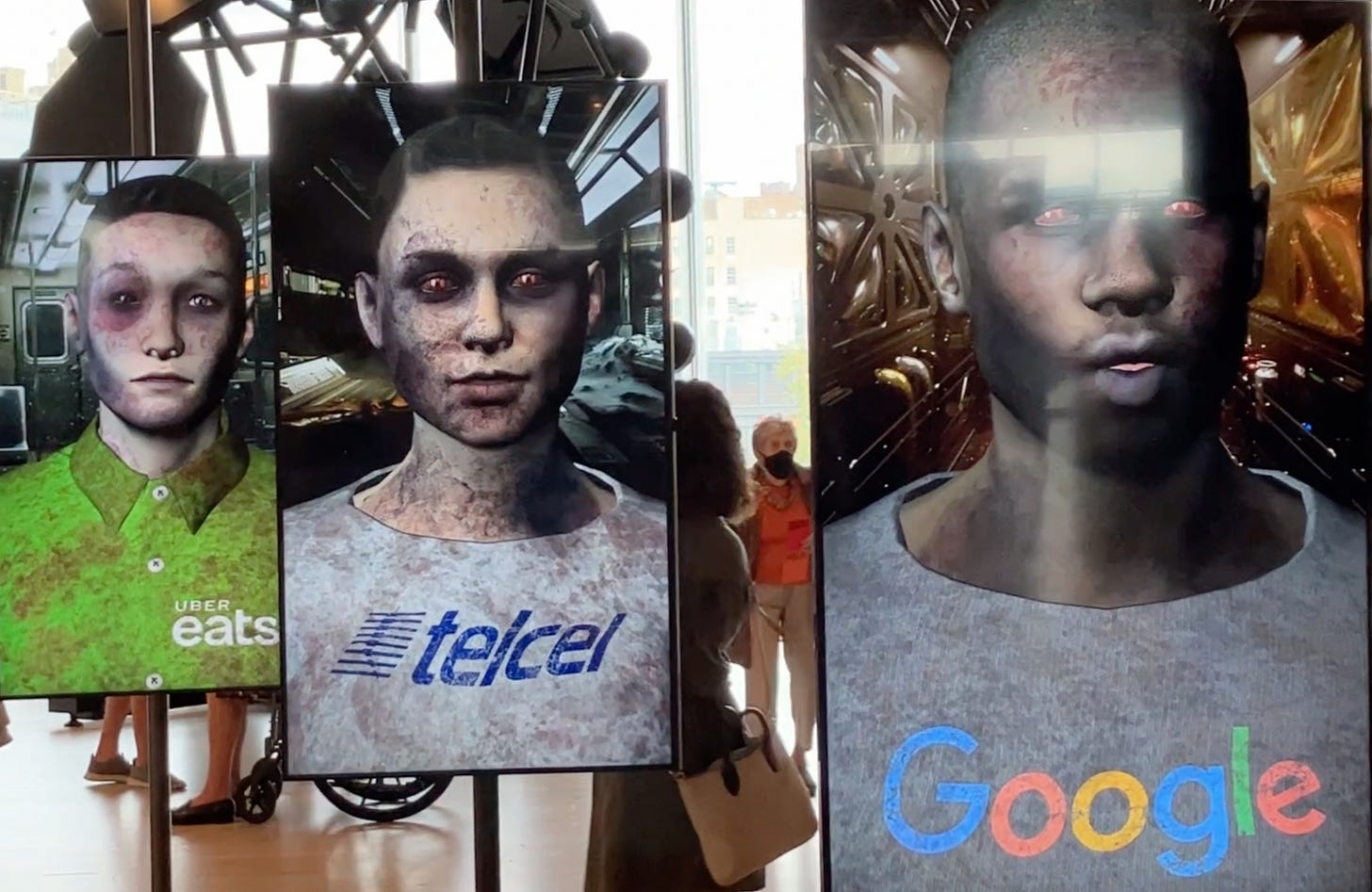
Teaching students nurtured on Google, it’s often worrying how weak their grasp of chronology is: the cybersphere may be geographically vast and marvelously interconnected, but it is happening in an eternal present. (Marina Warner, NY Review of Books, June 23, 2022)
The long bet
And there you have it. Kevin Buzzard had told me, and I had taken his word for it and repeated, that Szegedy wants to “put us [mathematicians] out of business.” Now I have finally found documentary proof that this is Szegedy’s intention and moreover that he is not only “fairly confident” but even confident enough to risk a small fortune on the fulfillment of his prediction.
The reference to 2029 seems to be a response to this extended post by Gary Marcus, which in turn was a response to Elon Musk’s two-line tweet predicting artificial general intelligence by 2029, and “Hopefully, people on Mars too.” But it also lines up with Szegedy’s 2019 prediction, quoted by Kevin Buzzard, and mentioned previously on Silicon Reckoner (and also here) that a “human level mathematical reasoning engine” was on the agenda for the “next decade.”
About that small fortune: Melanie Mitchell was apparently the first to suggest a long bet in response to Musk’s tweet. Mitchell, and perhaps Musk as well, were thinking of the 2002 long bet
By 2029 no computer - or "machine intelligence" - will have passed the Turing Test.
predicted by Mitchell Kapoor and challenged by Ray Kurzweil. In those days, when Facebook didn’t yet exist and even Jeff Bezos was only worth a paltry $1500000000, the $20000 stakes may have seemed like real money. But by 2022 Marcus was offering to put up $100000 and within a day was joined by like-thinking colleagues with a total of half a million dollars to burn.
Nearly four months have gone by and Musk has still not replied. Just like any other Martian visitor, I find this sequence of events and tweets (is a tweet an event?) curious and worthy of comment:
There are sharp disagreements about the timeline for AGI. Students nurtured on Google may be living in an “eternal present,” as Marina Warner observes, but the AGM (that’s Artificial General Mathematician to you) crowd, up to and including a fair cross-section of Google engineers, has been just 10 years away from success ever since the 1950s, a fact that was inevitably pointed out by one respondent to Marcus’s tweet.
But you have to be rich if you want your perspective to be taken seriously. “56% of Americans can’t cover a $1,000 emergency expense with savings,” according to a January 2022 CNBC headline, and you can bet that neither Musk nor Marcus nor Kapoor nor Kurzweil will be inviting anyone from this silent majority to weigh in on AGI. I am fortunately in the 44% minority but I’d already feel uncomfortable at the $100 gaming table.
And it’s not clear that those who hope to patent the first AGMs are terribly interested in communicating with human mathematicians either. When challenged to make his prediction precise, Szegedy tweeted anew:
As of June 30, Google engineers are already making claims about the first part of Szegedy’s AGM test.
Lewkowycz et al. are not (yet) talking about putting us out of business:
The model’s performance is still well below human performance, and furthermore, we do not have an automatic way of verifying the correctness of its outputs. If these issues could be solved, we expect the impacts of this model to be broadly positive. A direct application
could be an accessible and affordable math tutor which could help improve educational inequalities.
And even if it doesn’t reduce (“improve”) inequality, I can easily imagine how it might disrupt (“improve”) education, universities included.
Mathematical reasoning for engineers
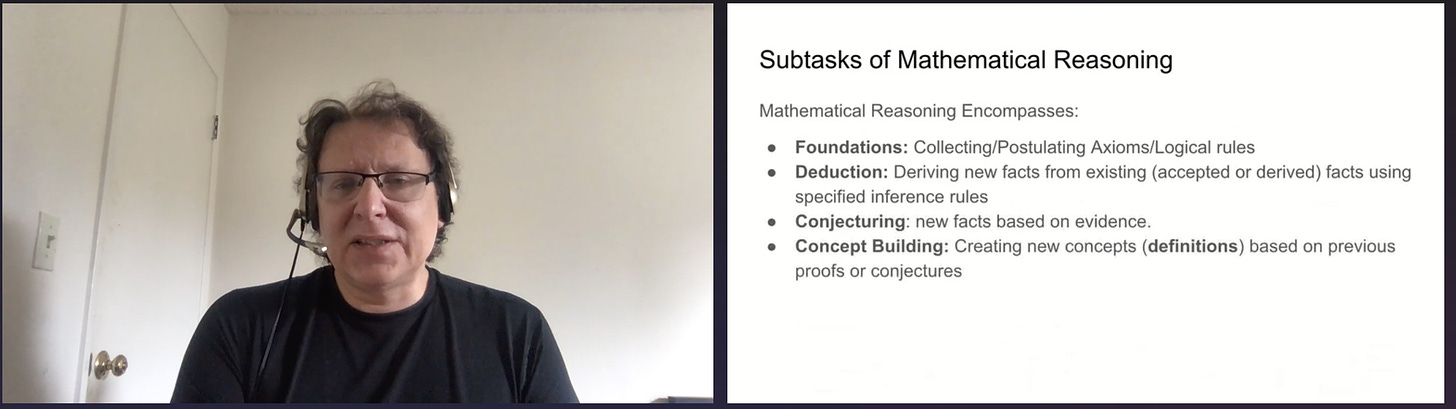
A competent engineer setting out to build a mechanical mathematician would begin by listing specifications. Szegedy’s breakdown of mathematical reasoning into “subtasks,” presented at an online symposium in December 2020, looks like the result of an engineer’s reflection on the problem, but the list is perfectly reasonable as a first description of the sort of thing mathematicians do when we do reason.
Szegedy acknowledges that establishing foundations “is done relatively infrequently, but has a high importance.” It seems that by “foundations” he means the metamathematical systems that are the subject of lively controversies in communities like the FOM list, in which case I disagree that they are of particular importance to mathematicians1 — it’s no accident that the FOM list is hosted by the NYU Computer Science department. Yuri I. Manin2 contrasted "foundations" of this sort
…the paraphilosophical preoccupation with nature, accessibility, and reliability of mathematical truth [or] a set of normative prescriptions like those advocated by finitists or formalists.
with his own use of the word
in a loose sense as a general term for the historically variable conglomerate of rules and principles used to organize the already existing and always being created anew body of mathematical knowledge of the relevant epoch.
This is the sense in which André Weil set out to establish the Foundations of Algebraic Geometry in a book published in 1946 and reprinted in 1962, the same year Grothendieck circulated his radically different Fondements de la géométrie algébrique. “Always being created anew” is the process described two weeks ago as the “search for the correct [or right] definition.” Szegedy includes this process not under “Foundations” but rather under “Concept building,” the last item on his list; he admits in the color-coded slide below that this is the “hardest open problem” for AI mathematics:
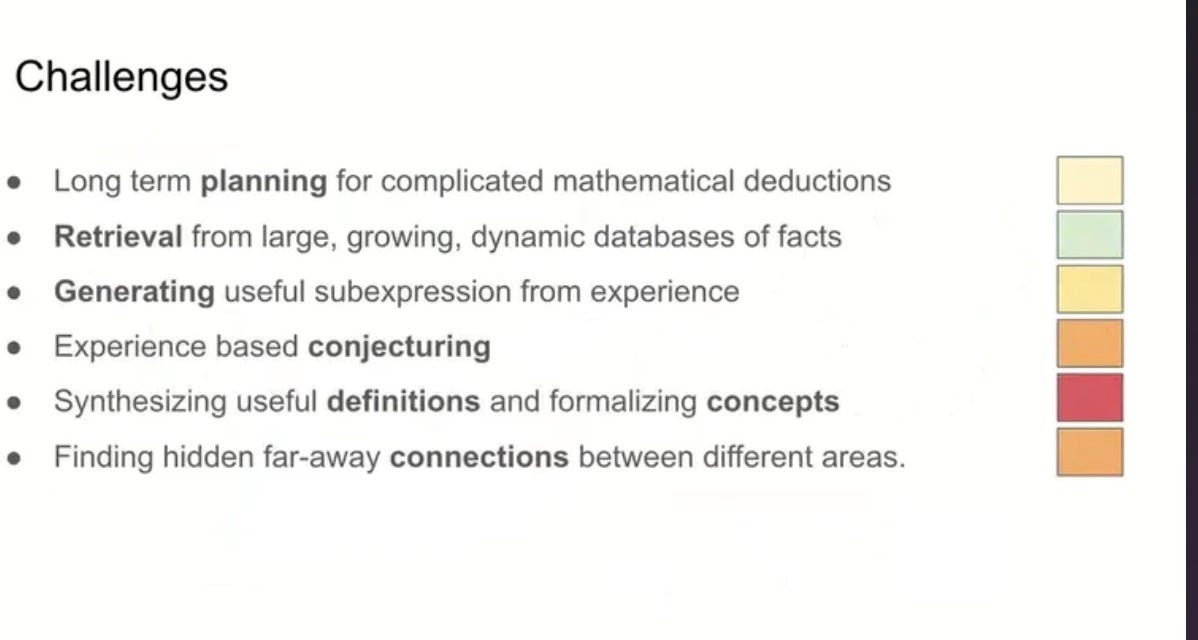
The “missing part,” Szegedy tells us, “is a good objective function,” a clear indication that he conceives what Scholze calls “find[ing] the right definitions” as the solution to an optimization problem, or more precisely on the model of a problem in machine learning.
Szegedy’s attention to concepts is something of a welcome surprise. There’s no more tired 21st century cliché than the claim that big data means “the end of theory.”
…in the era of big data, more isn't just more. More is different.3
The word “concept” is native to philosophy if it’s native anywhere, yet philosophers have never come to a consensus on what a concept is; which has led some philosophers to propose a philosophy and science without concepts. And big data is supposed to make this possible. Yet concept eliminativism doesn’t seem to have many adherents among AI researchers. At least the word still has a privileged place in AI discourse. If that weren’t the case, how could a Google research team make the announcement (that many of you probably remember) that their network of 16000 computers “basically invented the concept of a cat”?
Concepts and pattern recognition
Art is dead, dude. It’s over. A.I. won. Humans lost. (Jason Allen, quoted in NY Times, September 2, 2022)
I may be reading too much into the red box Szegedy placed next to “synthesizing useful definitions and formalizing concepts”. Perhaps the challenge refers not to the words in boldface but to the adjacent words: “useful” and “formalizing.” If what you and I think of as a concept is just an eigenvector of a covariance matrix or a principal axis of a high-dimensional scatter plot — which must have been roughly how Google’s 16000 computers represented their “concept of a cat” — Szegedy’s challenge is practical rather than ontological. Concepts did not guide deep learning’s sudden improvement at tasks like speech recognition, cat recognition, or automatic translation (between two languages for which adequate data is available). What AlphaZero has, when it wins at Go, is not an explicit elaborated strategy but rather a “good objective function,” the clearly defined objective being to win.
A “useful” mathematical definition, in the engineer’s mind, would correspond to winning. In principle this is not so different from what Scholze said about finding the right definition, since mathematicians also have objectives. The divide in the AI community between optimists and skeptics, if there is one, may parallel the classic distinction between empiricists, for whom winning amounts to the proper organization of masses of data, and rationalists who see concepts as indispensable. The divide is parallel to the one between philosophers who find John Searle’s Chinese Room argument convincing and those who see it as a gimmick. Thus Gary Marcus, a prominent skeptic, is not convinced by automatic text generators:
Clearly, knowing some statistical properties of how words are used in a concept is not sufficient for understanding the nature of the underlying concepts, such as what makes an airplane an airplane rather than a dragon (and vice versa)… In essence, GPT-2 predicts the properties of sequences of words as game tokens, without a clue as to the underlying concepts being referenced.
About a year ago I admitted to being baffled by Szegedy’s proposal to program automatic formalization of informal mathematics which is “given as a picture.” A proof in formal mathematics is “about” nothing more or less than signs on the page. This is what Hilbert meant when he argued that geometry could just as well be “about” tables, chairs, and beer-mugs, provided their formal relations were equivalent to those between points, lines, and planes. I can’t be sure I’m interpreting Szegedy correctly, but if his autoformalization machine is really learning mathematics by scanning images of mathematical text, then his mathematics is not “about” anything, and concepts as such are perhaps only a handy descriptive device.
This leads to what seems to me to be the crucial
Question: Does an AI need “aboutness” in order to display “human-level” intelligence? Or should humans scrap “aboutness” in order to acquire AI-level intelligence?
On the latter hypothesis, the above image of Confucius at the Women’s March, created by scraping the web and visibly not “about” anything, would be a successful demonstration of the level of creative intelligence expected of a future human-AI convergence.4
100 preselected human conjectures
I have to fight the temptation to write a flippant response to Szegedy’s prediction that an AI will “completely autonomously” solve 10% out of a list of 100 preselected human conjectures. Depending on how big a conjecture has to be, it would be easy for any mathematician to write down a list of 100 conjectures with total confidence that no AI will solve any of them in the foreseeable future. Does the Hodge conjecture for hypersurfaces of degree 23, 24, and 25 in in 9- and 11-dimensional space count as six, or three, or two, conjectures? Or as one conjecture, because they were written within the grammar of a single sentence? Or as an infinitesimal fraction of the Clay Millenium Problem called simply “The Hodge Conjecture”? The Twin Prime conjecture gets all the attention, but it is also conjectured that, for any even number 2k, there are infinitely many pairs of primes that differ by 2k. Is that one conjecture or infinitely many conjectures?
The reason I could express “total confidence” just now is that I am convinced that any conjecture whose solution would impress mathematicians in my area would require the development of new concepts, and in December 2020 Szegedy agreed that this is the “hardest open problem.” If less than two years later Szegedy was willing to commit to a long bet on solving 10 open conjectures (presumably “preselected” by an impartial committee) then either he believes this hardest problem will have been solved in time for the 2029 deadline, or he believes that concepts are not necessary to prove conjectures of any level of difficulty. The latter option would come as a surprise to Emmy Noether and her school of “conceptual mathematics” [begriffliche Mathematik],5 and since this vision of mathematics is currently inseparable from
…the goal of mathematics … to obtain a conceptual understanding of mathematical phenomena, and a deep understanding at that…6
we need not take it seriously.
I suppose there’s a third option — that an AI will by 2029 be able to juggle currently available concepts so much better than humans that 10% of preselected conjectures will just fall out in ways we could have foreseen if we were as “strong” as computers. This option sees the AI of the future handling mathematical concepts analogously to the way AlphaZero handled the rules of Go. AlphaZero invented no new rules; it just saw things in the existing rules that humans were too dim to perceive. However, the line that distinguishes new concepts from new ways to manipulate existing concepts is so fine that I’m just going to use my authorial prerogative to erase it for the purposes of this discussion. Thus I’ll assume that Szegedy expects the “hardest open problem” to be solved, or at least 10% solved, in the next seven years, and is willing to bet real money in support of this expectation.
There is also the question of what is Szegedy means by “completely autonomously.” The AlphaZero precedent suggests that the AI would be given nothing but the “rules” of mathematics — could this mean the Zermelo-Fraenkel axioms? — and the list of 100 preselected conjectures, and would then “play against itself” quadrillions of times, recording the conjectures on the list as they fall out. This looks to me guaranteed to trigger the kind of “combinatorial explosion” that Gowers’s “human-oriented” approach is designed to avoid. Szegedy addressed this issue briefly in his December 2020 slide presentation (slides 199 and 200):
…we train a two-stream neural network of two inputs: a goal and a potential premise. The goal is the one you want to prove, and the potential premise is one arbitrary premise from the database. And then we run it through some embedding network… and then we try to compute a rank, which ideally should be a probability that the potential premise is useful for the goal. In order to do that we concatenate the two embeddings and then run it through a combiner network. In order to make this practical… we don’t want to compute the whole network for every million premise and for each goal. So the idea is … we precompute the embedding for each of the potential premises and then compute the left part of the network only [see slide below]… most mathematical reasoning problems use more like a multi-layer perceptron that is relatively small and fast to compute… Of course there is the possibility that one creates a huge [proof-search] tree and a combinatorial explosion makes it impossible to prove the theorem…
However,
…if one has a very efficient premise selection and rule-selection network then the proof search can be efficient. And this is the way most neural theorem provers work now.
Szegedy hinted at what he means by “very efficient premise selection,” and presumably what 18 months later he thought will be necessary to meet the 2029 deadline, when he mentioned the use of ranking networks in “various domains” including “web search or… object recognition.”
This seems to bring us back to the “concept of a cat” that Google invented 10 years ago. But Szegedy’s presentation doesn’t contain enough that is recognizably mathematical for me to judge the likelihood that his “very efficient” networks can forestall combinatorial explosion, or for that matter to evaluate the existential risk his program represents for our profession.
I’m reluctantly coming to the conclusion that a tweet, or even a series of two tweets, is not an adequate medium to convey anything approximating a precise intellectual program, and that a 40-minute slide presentation isn’t much better. On the other hand, tweeting is a very effective way to communicate one’s intention to place one’s money at risk in support of an idea. Putting those two thoughts together leads to the conclusion that betting, even betting real money, is no substitute for an argument. So, this Martian would like to know, why do human academics do it?
I am still of the opinion that a computer is a tool. Meaning that computers don't get in the way of people — it's people who get in the way of themselves by using this tool. If you give a man a saw, he can cut firewood quickly, or he can cut off his own finger. If he saws off his finger, it's not the saw’s fault.
The same thing applies to artificial intelligence. (Maryna Viazovska, 2022)
Maryna Viazovska is not entertaining the possibility that the AI sees people as tools. Allowing that possibility is contrary to Kantian ethics, which is perhaps the starting point for a prominent strand7 in AI research, though not the only strand, as the above quotation from Joscha Bach (found in the disorder Marcus's long bet offer provoked in the twittersphere) demonstrates.8
I would like readers to consider the possibility that talk of “putting mathematicians out of business” amounts precisely to treating people — mathematicians, in this case — retrospectively as tools, for some purpose that tends to be poorly defined. This claim can of course be misconstrued, so I advise readers to recall how Donald MacKenzie, writing of early predictions for the future of AI, described the ability to prove theorems as part of “the essence of humanness.”9 The implication is that this ability is inseparable from being human in a way that using human muscles to move massive blocks of stone — like those used to construct the Egyptian pyramids, or Stonehenge — presumably is not. I don't claim that there is consensus on this "essence." Perhaps the essence of humanness is not to have an essence beyond not being a tool.
Logicians excepted; but most of the logicians I know don’t claim that work on foundations is of special importance to mathematicians in other fields.
in Mathematics as Metaphor, p. 49. See also his discussion in “Foundations as Superstructure”:
At each turn of history, foundations crystallize the accepted norms of interpersonal and intergenerational transfer and justification of mathematical knowledge.
There will have to be more about “about” and its metaphysics in a future installment.
According to Alexandrov’s obituary of Noether, this was the “mathematical doctrine” of which she was “the most consistent and prominent representative.” Van der Waerden’s obituary (Nachruf, Mathematische Annalen 111, 1935) was more expansive on this point:
Die Maxime, von der sich Emmy Noether immer hat leiten lassen, könnte man
folgendermaßen formulieren: Alle Beziehungen zwischen Zahlen, Funktionen und
Operationen werden erst dann durchsichtig, verallgemeinerungsfähig und wirklich
fruchtbar, wenn sie von ihren besonderen Objekten losgelöst und auf allgemeine
begriffliche Zusammenhänge zurückgeführt sind.
I thank David E. Rowe for this reference, which was cited in the play Diving into Math with Emmy Noether (see note 6).
This is a quotation from Jeremy Avigad’s article “Varieties of Mathematical Understanding,” in which he traces “the conceptual view of mathematics” from Noether through Dedekind back to Gauss. Avigad’s article sets out to “consider[] ways that recent uses of computers in mathematics challenge contemporary views” — specifically what he calls the conceptual view — “on the nature of mathematical understanding.” I still intend to devote a future essay to Avigad’s reading of the role of conceptual understanding and how it differs, subtly but dramatically, from the interpretation of Herbert Mehrtens, for whom the concept in “modern” mathematics is “simultaneously the object and instrument of investigation” [Untersuchungsgegenstand und Instrument der Untersuchung gleichermaßen]. (Mehrtens’s own sentence is too sibylline to cite here; I quote from its exegesis in an article of Mechthild Koreuber entitled Zur Einführung einer begrifflichen Perspektive in die Mathematik: Dedekind, Noether, van der Waerden.) Avigad, in contrast, suggests that “It may be helpful to think of conceptual mathematics as a technology that is designed to extend our cognitive reach” — in other words, that the concept is the instrument and the object is to be sought elsewhere. The historians can sort out which version more closely corresponds to the positions of Dedekind and Noether; but even if they rule in favor of Avigad I think his sentence is not the message we should be sending to engineers like Szegedy.
This long footnote was inspired by my attending Diving into Math with Emmy Noether in its final U.S. performance — but I encourage you to catch it when it comes back next year!
See the review on this site of Stuart Russell’s Human Compatible as well as Brian Christian’s The Alignment Problem. Both titles express the conception of humans as the end of AI rather than the means.
In a brief 2018 video, Joscha Bach discusses the origin of human ethics, then immediately and charmingly adds (at 3'27") “These constraints don’t apply to AI.”
See the discussion around footnote 3 in “Can mathematics be done by machine? I”.
The fact that AlphaZero was trained without using any pre-existing human chess games (or games played by other chess engines) seems of less importance than the fact that it taught human beings new techniques - something about how to put pressure on individual pawns, I heard. Any method to produce new techniques that humans can learn and use is interesting, regardless of whether a human or computer did it, and regardless of how much existing knowledge was or wasn't fed in.
In math, rather than arguing over what conjectures are too big or small to include on the list or the plausibility of computers proving conjectures without prior input, it seems better to formulate problems that, if solved by computers, would constitute computers helping human mathematicians in some way, for example by teaching us new techniques.
One could carefully select conjectures that the greatest number of mathematicians would like to cite a proof of but the least number of mathematicians want to prove or one could literally try to get someone to bet on a challenge like "10 new entries are added to Tricki describing tricks of a similar nature to the existing entries developed by AI mathematicians" or "AI programs a virtual reality system that allows humans to prove the geometrization conjecture visually." Why not?