A Gowers Manifesto
The brain's "hardware" and other metaphors are irrelevant to the manifesto's message.
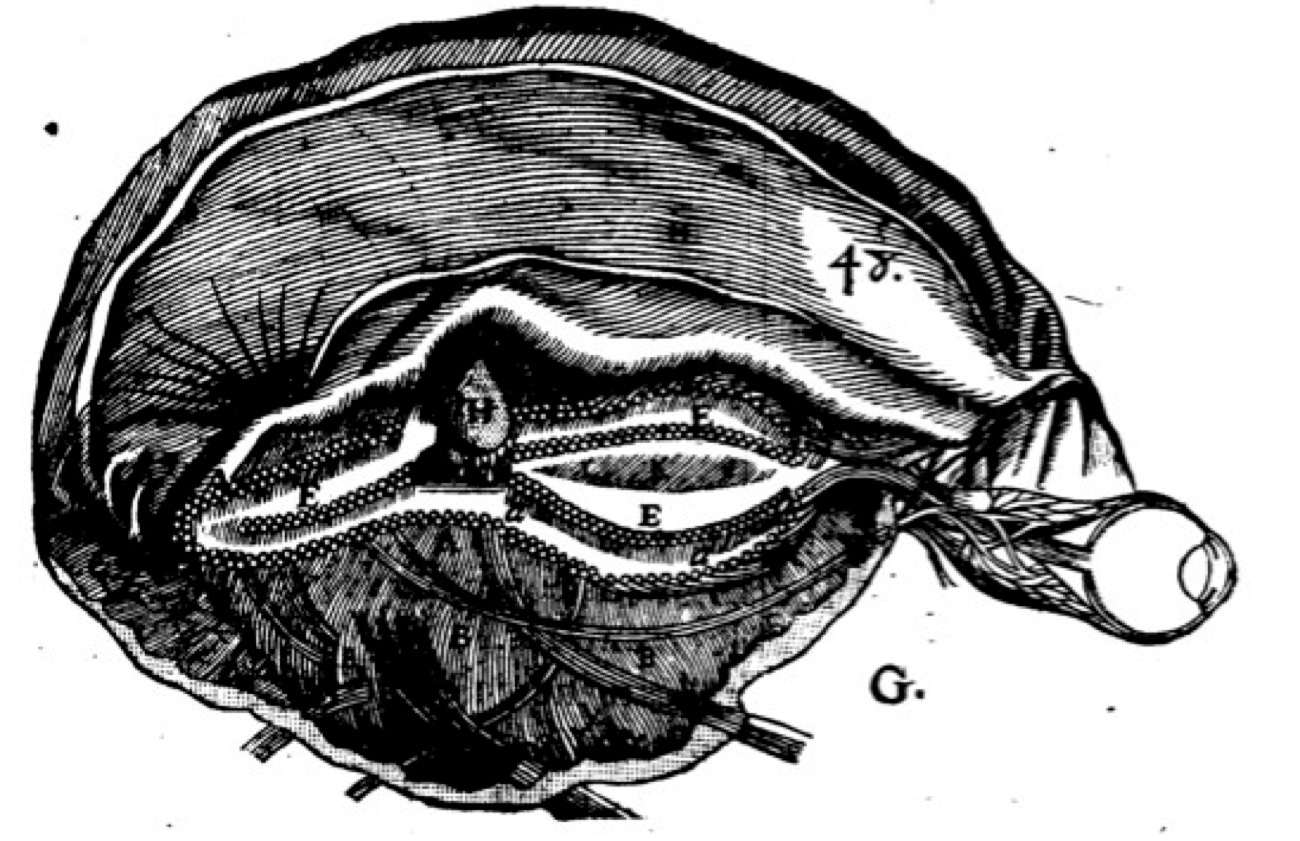
It is not [the figures] imprinted on the external sense organs, or on the internal surface of the brain, which should be taken to be ideas—but only those which are traced in the spirits on the surface of the gland H (where the seat of the imagination and the ‘common’ sense is located). That is to say, it is only the latter figures which should be taken to be the forms or images which the rational soul united to this machine will consider directly when it imagines some object or perceives it by the senses.
(Descartes, Oeuvres complètes, XI:176)
[By mistake I checked a box that allowed Substack to place a “subscribe” button in this spot. This unfortunately went out with the email to subscribers, to whom I apologize.]
Descartes’s “gland H” is, of course, the pineal gland, illustrated in the above sketch of the human brain. Everyone knows Descartes was a dualist, but in the text quoted above he was seeking a materialist explanation of ideas, regarding which he wrote
I wish to apply the term ‘idea’ generally to all the impressions which the spirits can receive as they leave gland H.
The “spirits” in question are “animal spirits,” spiritus animalis, a notion Descartes borrowed from the scholastics but reimagined as a material composed of “les plus vives et plus subtiles parties du sang que la chaleur a raréfiées dans le cœur1”:
La genèse des esprits animaux, telle que la comprend Descartes, n'est pas compliquée. Il se développe continuellement dans le cœur une chaleur vive, qui fait circuler le sang dans tous les vaisseaux. Grâce à elle, le sang le plus pur dépose sans cesse dans les cavités dn cerveau, où il se rend en ligne droite, les parties les plus vives et les plus subtiles de sa substance, et, sans subir de changement subséquent, ces parties sanguines prennent le nom d'esprits animaux.2
Descartes’ picture of the material basis of ideas was inspired, according to the Stanford Encyclopedia article, by then-recent advances in neuroanatomy, and is hydraulic rather than information-theoretic in nature. Memory is the effect of animal spirits sloshing around “the cavities and pores of the brain”:
when the soul wants to remember something, this volition makes the gland lean first to one side and then to another, thus driving the spirits towards different regions of the brain until they come upon the one containing traces left by the object we want to remember.3
Electronic metaphors
Hydraulic metaphors have long been out of fashion where thinking is concerned. The invention of the telephone and the rise of electrification subordinated the brain to a new collection of metaphors.
The analogy between these electrical organizations, and the higher systems of animal life is most striking. Comparing them with the human body, we may regard the heart as the battery or machine generating the vital fluid, the arteries as the outward conductor, and the veins as the return circuit; the brain and nervous system may be called the transmitting or manipulating devices, and the senses, and the organs by which they are manifested, the receiving instruments.4
Contemporary accounts of brain function are expressed in yet a different vocabulary, involving electrical signals and neurotransmitters, notions barely familiar to the author of the above 19th century passage, and completely unknown to Descartes, who did his best with the vocabulary that was available at the time. We like to think that because they have the scientific method our scientists are working with the final and optimal model — whatever the topic — and that only the details of its functioning need to be filled in. So it is helpful to remember that Descartes thought the same about his model less than 400 years ago, a negligeable interval on the scale of geological time. I have no doubt that neurons and so on correspond to real physical structures and functions in the brain and that the half-life of today’s neuron theory will far exceed that of the theory of animal spirits.5 It lends itself a bit too neatly, however, to modeling by the kinds of electronic circuits invented for the purpose of building digital computers to embody existing theory, and thus provides unwarranted support for the metaphor of the brain as a wet and sloppy Turing machine. That is what Tim Gowers has in mind when he refers to the brain as “hardware” in an article introducing his new project for automated theorem proving:
Of course our brains are complicated, but that is just the hardware, and my own experience of solving mathematical problems strongly suggests that I am using that hardware to run a much simpler piece of software that can be examined and understood.
(Gowers, “How can it be feasible to find proofs?”)
Later Gowers writes
I can regard myself as a kind of computer – and here I do not mean a massively complicated computer with neurons firing all over the place, but rather an extremely limited computer that is simulated by that much more complicated one (a bit like the virtual pocket calculator that I can get to appear on the screen of my laptop).
If this metaphor were doing any work in Gowers’s article it would be to promote the cognitivist perspective:
The assumption that the ‘software’ of cognition could be studied independently of the ‘hardware’ has had a marked impact on the field. Whereas before the cognitive revolution systems of perception and action held a central place in explaining human behavior, after the cognitive revolution these systems became irrelevant to the study of cognition.6
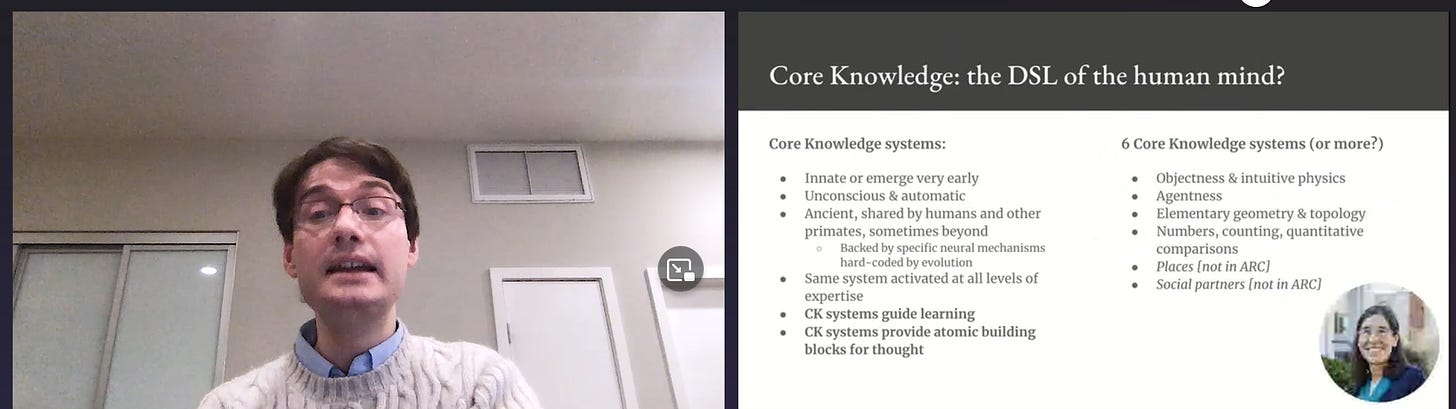
In practice this is often a sensible attitude. Most of us don’t look under the hood when we drive a new car; we don’t learn the operating system before switching on a new smartphone; we don’t bring along a PET scanner on a first date. If and when it gets to the point where we suspect that some of our colleagues are robots, we’re more likely to administer a Turing test7 than to open them up with a scalpel.
But metaphors guide us when we are trying to develop new ideas, and misguided metaphors are likely to misguide us. Cognitivism, with its emphasis on mental processes, arose in part as a healthy reaction to behaviorism (and in part as an embrace of the theory of mind that made electronic computing possible). But with its identification of thinking with problem solving it has come in for a fair share of criticism from a variety of quarters, and no longer enjoys the prestige among philosophers that it did 50 years ago.
The Gowers Manifesto
Fortunately, while the above passage of Gowers’s article may well reveal a bias toward cognitivism — shared, perhaps, with many of the computer scientists who are working to automate mathematics — it has no bearing on the article’s substance. Gowers has been speculating as seriously as anyone about future interactions between human and mechanical mathematicians at least since the fateful year 1999, when his talk at the Visions of Mathematics conference imagined “a dialogue between a mathematician and a computer8 in two or three decades’ time.” And for nearly half of that time, he has gone well beyond speculation, devoting considerable effort with successive collaborators — Mohan Ganesalingam, Ed Ayers and Bhavik Mehta — to ensuring that the dialogue will be on human rather than mechanical terms.
Gowers’s article was announced in a lengthy blog post in late April and was already quoted briefly in an earlier installment. It’s a sign of the significance he attaches to this 54-page article that Gowers gave the title manifesto.pdf to its file. Mathematics has generated other manifestos in recent years, devoted to mathematics teaching (see also this manifesto at Gowers’s home university) or to rethinking gender, but manifestos on the conduct of mathematical research seem to specialize in the implications of mechanization, starting with the QED Manifesto, released in 1994 and the subject of its own Wikipedia page.
The QED Manifesto, though authored anonymously, was (manifestly) the work of computer scientists, and it should by no means be assumed that they share the values that motivate mathematicians. Only an ideologue, or a computer scientist, could imagine that Freek Wiedijk’s vision of
would appeal to mathematicians. The sympathy for machines manifested here and in Gowers’s manifesto contrast sharply with the outlook of a much earlier but better-known manifesto:
Owing to the extensive use of machinery, and to the division of labour, the work of the proletarians has lost all individual character, and, consequently, all charm for the workman.… in proportion as the use of machinery and division of labour increases, in the same proportion the burden of toil also increases…
Gowers is a mathematician, of course, and not an ideologue. His perspective is cognitivist only insofar as it aims to understand the "software" at the heart of human mathematics well enough to run it on machines. His vision of "extreme human-oriented automatic theorem proving" (EHOATP) is one that "does not cut corners by using computery methods such as brute-force searches, even when those searches are feasible." This approach has a theoretical justification: finding proofs of fixed length by brute-force search is an NP-complete problem, while finding proofs with no restriction on the length is undecidable. (In order to reason in this way, a "proof" must necessarily be a formal object, in keeping with the Central Dogma.) "And yet," he writes, "human mathematicians have considerable success with solving pretty complicated looking instances of this problem. How can this be?"
Methodological concerns
To resolve this conundrum Gowers posits that the objects to which the Central Dogma pertains — pairs (S,P) with S a well-formed mathematical statement and P a formal proof of S — form a set called B (for “boring”) that is much larger than its subset M (for “mathematics”) that is relevant to mathematicians. He then formulates his first methodological question:
…for a complete understanding of how it can be that humans find proofs, one would like good answers to the following two further questions.
1. What distinguishes the pairs in M from general pairs in B?
2. Why is it frequently feasible for humans to find a proof P of a statement S when the pair (S, P ) belongs to M?
With this in mind, Gowers begins, in a section entitled "What this project is not," to draw distinctions from other paradigms. Thus this is not part of his project:
I take the view that [formalization] is not a prerequisite, and that there is no reason in principle that a computer couldn’t find proofs that are not fully formalized but that can be informally verified (that is, seen to be correct) by humans.
Nor does his project overlap with the machine-learning paradigm:
The ideal, for a machine-learning researcher, would be a system that can look at a large number of existing proofs and, with no human intervention at all, learn for itself what proofs are and how to find them.
We have already explained our reasons to be skeptical about such an approach, as it is being pursued by Christian Szegedy's team at Google Research. Gowers's skepticism, as you might have expected, is more polite than mine:
…humans do learn to find proofs, but they do not do so by simply looking at a huge number of proofs and “getting the idea.”
And we have already seen that he doesn't want to follow the "machine-oriented approach" to automated theorem proving that makes use of the capabilities that machines enjoy — are we ready to call them "talents"? — and that we humans do not. Instead he bets that "there is a lot of mileage still" in GOFAI ("good old-fashioned AI"), enough to build his EHOATP, by building on human mathematical common sense:
by examining very carefully how humans discover proofs, one trie[s] to understand the process well enough to be able to program it.
Why human-oriented?
Gowers’s commitment to the extreme human orientation is not merely sentimental. There are practical reasons to avoid relying on the computer’s unique abilities; proof search based on raw computing power quickly becomes intractable:
the extreme human-oriented approach …[or] something like it is needed if one wants to avoid a combinatorial explosion later.
A second reason for advocating EHOATP is more optimistic:
…if we understand how to avoid search for very basic problems, we will uncover principles that will help us understand how we avoid search for less basic ones. This conviction depends on the idea, which is not necessarily correct … that the question “What is it about M that makes it tractable?” has some sort of unified answer.
Gowers’s dream is that this answer might take the form of a precise theorem:
a proof is easily generated if it is the output of an algorithm that has access to a certain body of background knowledge, takes as its input the statement to be proved, and is heavily restricted in certain ways yet to be worked out. The dream would then be that there is a non-trivial theorem that shows that if a proof is easily generated, then it is also easy to find. This might seem to go against the almost universally held belief that P̸=NP – finding should be harder than checking – but here I allow the algorithm that finds the proof to be quite a lot more powerful than the one that generates the proof.
Teaching machines to be human
Gowers allows himself to dream but his actual project is refreshingly modest. He is clear-eyed in his acknowledgment of the limitations of current methods, including his own. His willingness to seek incremental progress provides a welcome contrast with the ambitions advertised by the foundation funding the project, the Astera Institute,9 that promises to “bring humanity the greatest imaginable good in the most efficient possible way” and in this way “to create transformative progress for human civilization.” For Gowers it would be transformative enough to train a computer to solve the
Problem. Prove that a uniform limit of continuous functions is continuous.
This basic theorem from undergraduate analysis stumped the system Gowers and Ganesalingam designed. Gowers has some ideas about why their system failed to find human-like proofs of this and a few other elementary facts, and he outlines them in the 25-page technical discussion that occupies roughly half of his manifesto. The importance of such problems for Gowers is that their solutions require moves that occur effortlessly to human mathematicians but that no automatic theorem proving approach has managed to emulate. Gowers believes that such moves provide a key to the practice of human mathematicians; with regard to the theorem on uniform convergence, he anticipates that
if one could solve this problem in a suitably general way, then one should be able to solve many other problems of comparable difficulty.
Gowers defines several kinds and degrees of success; they have in common that they require the machine to do something that hasn’t been done before, and he is careful to be as concrete as possible about what this would entail. He specifically rules out success that involves what he calls “cheating”:
it would be good to have a formal definition of cheating, so that one could say, “We have found an algorithm that solves this problem and here is a proof that the algorithm doesn’t cheat.”
Absent such a formal definition, Gowers resorts to identifying different sorts of cheating that share what one might call a Wittgensteinian “family resemblance.” And he doesn’t want this condition to be too constraining. After setting himself an artificial problem about Fibonacci numbers, and proceeding to solve it by a few clever tricks, he argues that
building [domain-specific tricks] in, though it is cheating in a way, is no more cheating than human mathematicians cheat when they are taught general tips by other mathematicians.
I can’t summarize the technical discussion here but I encourage readers to study this part of the manifesto in particular. None of the other teams that seek to endow AI with the magic of “human-level mathematical reasoning” has thought nearly as deeply and insightfully about what humans actually do. The effort that has gone into identifying the goals of the project, and that will undoubtedly continue to refine these goals, already makes it important to follow, whether or not these goals are realized.
It would take much more than finding solutions to well-formulated problems, however, to convince me that what Gowers calls “the grand goal of the area… to create a computer program that is as good … as a research mathematician” in proving theorems, is at all realistic. To qualify as “human-level” the computer would (at least) have to choose its own problems. But that’s a topic for a future post.
Intended or unintended consequences?
Les choses fonctionnent bien, tout le monde en est content, elles sont conçues avec bon sens et solidité — et il faut qu’ils démolissent ce qui convenait pour monter à la place des machins auxquels personne ne comprend rien.
(Virginie Despentes, Vernon Subutex, book 2)
I mentioned that Gowers doesn't see formalization as a prerequisite to his project. But he does predict that future mathematics will necessarily be formalized:
much of this low-level work will at some point be automated, and … higher-level and more mathematician-friendly languages will be developed that can be used to write the proofs that computers can verify. Once this happens, it is likely that there will be a cultural shift, and that what mathematicians understand by “proof” will be more like “formally verified proof” than the arguments we produce today, which we fondly and not always correctly assume to be formally verifiable at least in principle.
Quite what the social consequences of building such a system would be is not clear, but similar considerations applied to calculators and society adjusted.
I'm not sure what he means by this last sentence. His allusion to calculators suggests that he may be thinking of the collapse of the slide-rule industry, which must have been studied somewhere in the economics literature. Or he may have in mind human calculators like the women celebrated in the film Hidden Figures, who went on to distinguished careers based on the skills they developed in getting the US space program literally off the ground. It's not clear to me why the particular precedent of the calculator is more pertinent to mathematical research than the invention of the typewriter.
A more apt example of "cultural shift" is the one in number theory that accompanied the invention of cohomology of groups. It is still barely possible, and in many ways enlightening, to learn class field theory without explicit reference to cohomology of groups. But it's futile to eschew the cohomological language if you want to work with class field theory, and in fact it takes a considerable effort to imagine what number theorists were thinking before the cohomological methods were available. The Birch-Swinnerton-Dyer Conjecture could not have been invented without the help of computers, but it's no accident that it was formulated in the language of Galois cohomology.
A more recent example is the reorganization of p-adic geometry, and much of arithmetic algebraic geometry, around the perfectoid methods introduced by Peter Scholze just over a decade ago. My next essay will explore how this and similar developments are recognized as "the right" way to approach mathematical problems. I have already alluded to the potential (but very real) "social consequence" that these new methods may leave me on the wrong side of a generational barrier.
This sort of thing happens all the time in mathematics. What the last two examples have in common — and I could mention many more examples — is that the new methods were adopted because they were aligned with the explicit priorities of the field. If my colleagues had hardware in their heads, instead of something that is frankly more akin to animal spirits, they might be clamoring as well for what the formalizers have to offer. But mostly they’re not, and I will explain in a future post why I don’t expect this to change.
Footnote: Google is my copilot?
Since I’m not in the habit of perusing back issues of The Electrician and Electrical Engineer it’s natural to wonder (it’s unnatural not to wonder) how I found that passage comparing the brain to a “transmitting device.” The secret is that I'm also a cyborg! With Google as my prosthesis I have a superhuman memory: I typed something like10 “brain” and “telephone circuit” in Google’s search box and this article was one of the top results.
How I got to this point has certain parallels with the search for proofs of theorems, and before that with the search for theorems to prove. The hapless programmers assigned to emulate my cerebral architecture will manage the “type into Google” algorithm easily enough but a wall of serendipity will confound their blueprints for the “type what?” module.
Descartes, “Les passions de l’âme,” 10.
A. Goffart, Les "Esprits animaux ". In: Revue néo-scolastique. 7ème année, n°26, 1900. pp. 153-172.
Descartes, “The passions of the soul,” 42; trans. Cottingham and Stoothoff.
Thomas D. Lockwood, “The Construction of Lines for Electrical Circuits,” The Electrician and Electrical Engineer, Volume 3 (July 1884) 143-4.
Actually, I wouldn’t be surprised if a kind of unnatural selection endowed people who think like machines with a reproductive advantage. But that’s a topic for another time.
R. A. Zwaan, M. Kaschak, “Language, Visual Cognition and Motor Action”, Encyclopedia of Language & Linguistics (Second Edition) (2006) 648-651.
or a Voight-Kampff test…
Identified with Klara 2.0 in this earlier essay.
Founded, as I’m sure you will be pleased to know, by yet another crypto billionaire.
That’s what I remember having typed, but repeating the experiment doesn’t return anything nearly as interesting, so maybe the event can’t be reproduced.